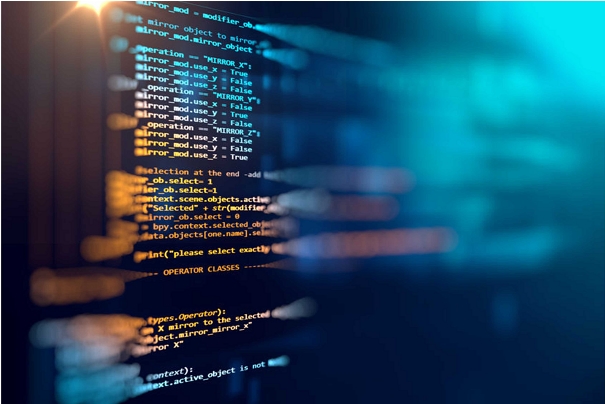

Academic and industrial researchers know full well that in order to be successful in science, you can't waste time on anything unnecessarily. This mantra includes meetings, grant-writing, publication, preparation of presentation materials, managing experiments in the lab, and analyzing data from experiments. With the ever-decreasing US NIH budget for medical research, in spite of being funded, most grants awarded today receive significant budget cuts which translate to dropping salary and fringe for a lab technician or dropping sub-aims of the research objectives that could potentially provide new insights into disease and establish new leads for future research. Altogether, there is an overwhelming sense of cost reduction (belt-tightening), increased efficiency and increased resource optimization in academic research.
Wasted functionality. In the early days of statistical software development (circa 1970s-1980s), software houses competed by offering more and more statistical tests, and features such as probit regression, power and sample size analysis, GLM, GEE, etc. The problem that ensued was that, over time, most of the large vendors programmed into their software literally everything they could "get their hands on" -- and their current customers are still paying for this unprecedented programming frenzy. The drawback of this "program everything" focus is that only a fraction of the software developed will ever be used. In short, most IT departments are likely wasting thousands of dollars per year for statistical software functionality which is never used because of developer over-programming.
A large challenge that software users face is hinged to the design concept for existing packages. These can be itemized as follows:
- Most software houses only develop a method from a one-dimensional perspective, as a means to an end for getting results to the user.
- What the user does with the results is the user's responsibility, not the developer's.
- Software design of computer-generated outputs are not linked to time efficiency and user productivity.
- The over-programmed software houses, who developed everything they could get their hands on in the 70's, 80's and 90's, are now stuck with millions of lines of legacy code which is rarely used -- but users still pay for this.
- The over-programming hasn't stopped: the introduction of new modules is ever-increasing in order to remain competitive.
Wasted time. There is also a good chance statistical software users are spending too much time to analyze data. Most packages require running a test for each pair of variables singly, and then manually transposing results (statistics and p-values) into Word, Excel, or PowerPoint presentations. So the problem is not only related to paying for features that will never be used, but also wasting precious time to create publishable results in grant applications, manuscripts, presentations, and research reports.
User-centric software challenges include:
- Software users only know what they have been taught in school, on the job, or from example runs in user guides, or blog posts.
- Example runs for tackling a problem are usually based on the already-suffering software issues enumerated above.
- Most users are unaware of the large amount of time they waste for performing routine analyses, including summarizing data, hypothesis testing, and model building.
New demands. Data analysis has also changed over the last few decades. Demand for software capable of data-driven analyses and text mining is now competing with the demand for software providing only hypothesis-driven statistical analyses, the latter of which involve the majority of large statistical software developer houses. The idea of "death of statistics" involving use of probability distributions to define everything is not a new one. In point of fact, most graduate students are now more interested in large-scale deep learning with artificial neural networks, or machine learning as a way of becoming competitive in today's employment markets.
Novel approach. NXG Logic's approach to software development starts with the realization of what most statistical software packages lack, namely, the ability to rapidly combine hypothesis test results for multiple variables into a single color-formatted output which could rapidly be pasted into manuscripts and presentations. In addition, there was a lack of more contemporary non-statistical methods. NXG Logic design concepts include machine learning, artificial neural networks, text mining, etc., and incorporate numerous time-saving steps so that the end-user can obtain more informative results faster, while optimizing research resources. Our development priorities since day one have always included:
- Machine learning techniques, generative and discriminitive modeling
- Swarm intelligence
- Class discovery and class prediction
- Super-resolution root MUSIC
- Component subtraction, decorrelating and denoising data
- Fast wavelet transforms, fuzzification
- Text mining and N-gram analysis
- Non-linear manifold learning and dimensionality reduction
- Hermite and Laguerre neural networks
NXG Logic also focuses on development of several fast-formatting technologies which combine output from runs made on multiple variables. These technologies include:
- PFA - Parallel Feature Analysis
- FFOSS - Fast Formatted Output for Summary Statistics
- FFOMT - Fast Formatted Output for Multiple Tests
- FFORM - Fast Formatted Output for Regression Models
- FFOA - Fast Formatted Output for Association
- FFOCD - Fast Formatted Output for Class Discovery
- FFOCP - Fast Formatted Output for Class Prediction
Using NXG Logic's Explorer package, researchers can generate results for more data in a fraction of the time required by most software packages. Whether it's text mining, machine learning, cluster analysis, ANOVA, class discovery, class prediction, predictive analytics, or survival analysis, Explorer can produce multi-variable results substantially faster and in a format that is much more informative when compared with most other packages.
The ChipST2C package (Chip Statistical Testing to Clustering) is a software package for RNA-Seq and DNA microarray data analysis. Capabilities of ChipST2C include 2- and k-sample parametric and non-parametric hypothesis testing, automatic hierarchical cluster analysis of statistically differentially significant genes, heat maps, k-means cluster analysis, principal components analysis (PCA), within-gene and between randomization tests, and various approaches for the multiple testing problem (Bonferroni, false discovery rate, and Storey q-values). In addition, K-means cluster analysis can be performed on significant genes for 2- and k-sample tests in order to drill down further into co-regulatory expression patterns.
The newly introduced NXG Logic Instructor package for learning/teaching biostatistics can substantially shorten the time required for generating statistical teaching materials, including homeworks, quizzes, exams, course packs, grading keys for TAs with worked solutions, etc. The rationale for developing the Instructor package was to reduce the time required for generating high-quality biostatistical teaching materials, including homeworks, quizzes, and examinations which could be randomly generated so that students have different parameters for questions and different simulated datasets. Student dishonesty and cheating is on the rise around the globe, and universities are constantly trying to increase their awareness of it while attempting to thwart its occurrence. By randomly generating quiz and examination questions with different parameters, and randomly generating different datasets for student projects, the Instructor package can be used to help overcome these issues.